In manufacturing, the use of log files combined with advanced technologies like Language Models (LLMs), Retrieval-Augmented Generation (RAG) applied at the edge represents a significant evolution in how root-cause analysis and risk assessment are conducted. This approach leverages detailed data recorded in real-time to provide a deeper understanding of operations, which is essential for identifying issues and preventing future problems.
The Importance of Log Files in Manufacturing
Log files, such as Equipment Automation logs and Equipment Data Analysis logs, record every action and event within manufacturing systems, capturing data on machine performance, system errors, operational deviations, and maintenance records. These files are critical for several reasons:
- Comprehensive Data Capture: Log files provide a comprehensive record of the operational state of machinery and processes. They capture detailed, time-stamped information that can be crucial for diagnosing problems after they have occurred.
- Historical Data for Analysis: For technologies like RAG, historical log files are invaluable. They allow the system to access past incidents, understand the context of those incidents, and apply learned solutions to current problems, making root-cause analysis more informed and effective.
Comparison with Traditional Methods
Traditional methods of problem-solving in manufacturing often involve manual checks and reactive measures after issues have already impacted production. In contrast, using log files with AI models and concepts (LLMs and RAG), applied in Edge AI technology offers several benefits:
- Proactivity: Log files enable a proactive approach. By continuously monitoring log data, Edge AI can detect anomalies early, often before they cause significant issues. This early detection is crucial for preventing downtime and ensuring product quality.
- Accuracy: Traditional methods can rely on human interpretation of symptoms, which might miss less obvious signs of problems or misinterpret data. Edge AI analyse log files with high precision, reducing human error and providing a more accurate diagnosis based on data trends and patterns.
- Speed: With traditional methods, diagnosing problems can be time-consuming, often taking place after a failure has occurred. LLMs and RAG analyse real-time data from log files instantly, speeding up the diagnosis and resolution process significantly.
- Cost-Effectiveness: Preventing failures and reducing downtime through effective use of log files not only saves direct repair costs but also minimizes production losses. The ability to quickly address issues before they escalate can result in substantial cost savings
Enhanced System Integration for Better Outcomes
Integrating LLMs and RAG to utilize log files effectively creates a robust system for manufacturing:
- Dynamic Risk Assessment: Using log files, Edge AI can continuously assess risks based on current and historical operational data. This ongoing assessment helps to implement timely preventative measures, minimizing risk exposure.
- Continuous Improvement: The feedback loop from continuous log file analysis allows systems to learn and improve over time. By analysing outcomes and interventions, the system adapts and becomes more effective at predicting and mitigating future issues.
- Streamlined Compliance and Reporting: Automated analysis of log files ensures compliance with regulatory standards by providing accurate records of operations and interventions. This streamlined reporting aids in compliance audits and operational reviews.
The Role of Edge AI in Industrial Manufacturing
Bringing LLMs and RAG to the edge enables localized processing by bringing intelligence closer to the source of data generation on the manufacturing floor. This offers several advantages:
- Real-Time Processing: Edge AI processes data at the source, enabling real-time analysis and decision-making. This immediacy is crucial for detecting and addressing issues as they arise, minimizing response times.
- Reduced Latency: By processing data locally rather than sending it to a centralized cloud, Edge AI reduces latency, ensuring that insights and actions are based on the most current data available.
- Enhanced Security: Keeping sensitive operational data on-site rather than transmitting it over networks enhances security and protects against data breaches.
- Scalability: Edge AI solutions can be scaled to meet the specific needs of different manufacturing environments, providing flexibility and adaptability to various operational scales.
Conclusion
The strategic use of log files in conjunction with Edge AI technologies transforms root-cause analysis and risk assessment in manufacturing. By enabling a proactive, precise, and efficient approach, this integration not only enhances operational reliability and product quality but also supports a culture of continuous improvement and innovation in manufacturing processes. The addition of Edge AI further amplifies these benefits by ensuring real-time, secure, and scalable data processing, making it an indispensable component of modern industrial manufacturing.
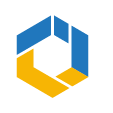
Edge AI Technology and the role of SoftwareCUBE
As a software development company, we experience constraints that come with using cloud-based AI, like delays, security risks and expensive operations. To address these issues our Edge AI initiative is dedicated to leading the way in cutting edge technologies for data processing at the network edge.
By looking at low power AI hardware and software infrastructure Edge AI aims to greatly improve effectiveness and safety while reducing the environmental impact of AI applications in various industries such as manufacturing, energy, healthcare and transportation.
We participate in the European Edge AI community and are partner in the EdgeAI project. Our aim is for edge AI technology and knowledge to result in innovative business prospects. We currently focus on increasing required data quality and completeness and designing AI based systems at the edge, which support anomaly detection and risk assessment.
EdgeAI “Edge AI Technologies for Optimised Performance Embedded Processing” project has received funding from Chips Joint Undertaking (Chips JU) under grant agreement No 101097300. The Chips JU receives support from the European Union’s Horizon Europe research and innovation program and Austria, Belgium, France, Greece, Italy, Latvia, Netherlands, Norway.